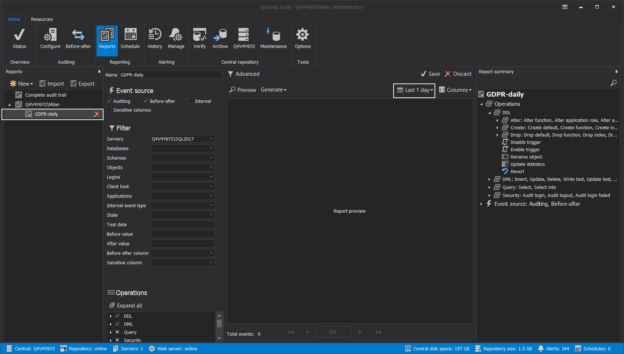
Introduction
In a scenario where SQL audit data requires special processing, reviews, and analysis, it can be accomplished by designing an in-house custom application to read the data and provide desired output and visualization. Since the ApexSQL Audit central repository database uses a proprietary design with encryption applied, a custom database will have to be used to provide data to the said in-house application.
December 15, 2021